Qdrant is an open-source and fully managed high-performance Vector Database that provides a production-ready service with a convenient API to store, search, and manage vector embeddings.
It is designed to support extended filtering, making it useful for neural-network or semantic-based matching, faceted search, and other applications. Qdrant is written in Rust, ensuring fast and reliable performance even under high load. It is also available as a fully managed Qdrant Cloud, including a free tier.
How does it work?
Qdrant supports advanced and high-performant vector similarity search technology, making it an enterprise-ready, high-performance, massive-scale Vector Database available as open-source, cloud, and managed on-premise solution.
It is designed to power the next generation of AI applications with its advanced vector search capabilities.
Qdrant offers a Python client library for easy integration into applications, and it also supports a wide range of client libraries for different programming languages. It is also available on Hugging Face, a popular platform for AI and machine learning models.
Qdrant’s vector search capabilities enable it to find similar images, detect duplicates, or even find a picture by text description. It also supports filters that enable arbitrary business logic on top of similarity search, making it a versatile tool for a wide range of applications.
How Does Qdrant Handle Data Privacy And Security?
Qdrant supports TLS encryption for all inbound and outbound emails, and uses Gmail for email and communication services. The location of data on Qdrant Cloud can be specified, with options including London, Ireland, Belgium, Germany, Switzerland, North America, South America, Australia, Canada, Tokyo, and Singapore.
Data will not be moved or replicated outside of a specified location. All data is encrypted when transmitted between client devices and Qdrant Cloud, and is encrypted at multiple levels when at rest.
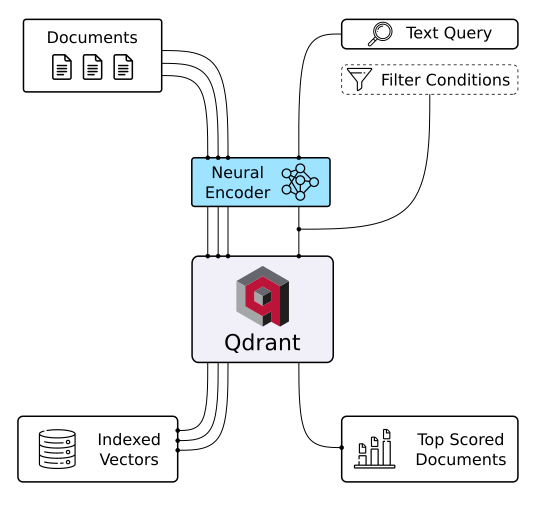
Auth tokens are stored using pbkdf2, bcrypt, scrypt, or argon2 hashing algorithms for added security. Qdrant Cloud services are hosted on Google Cloud Computing and Amazon Web Services, with data centers staffed 24x7x365 by security guards and access authorized strictly on a least privileged basis.
The cloud hosting providers are certified with the ISO 9001:2008, SOC 1, SOC 2, and SOC 3 standards. Qdrant takes a responsible approach to reporting and addressing security vulnerabilities, encouraging responsible disclosure and prioritizing the safety and security of their service.
Qdrant Cloud is compliant with the PCI DSS Level 4 requirements for security management, policies, procedures, network architecture, software design, and other critical protective measures.
Qdrant ensures that data is collected and processed in compliance with German law and the provisions of the Data Protection Laws.
And provides end-users with the right to access, rectify, delete, limit or oppose the processing of their data, as well as the right to define guidelines for the storage, deletion, and communication of their data after their death and the right to data portability.
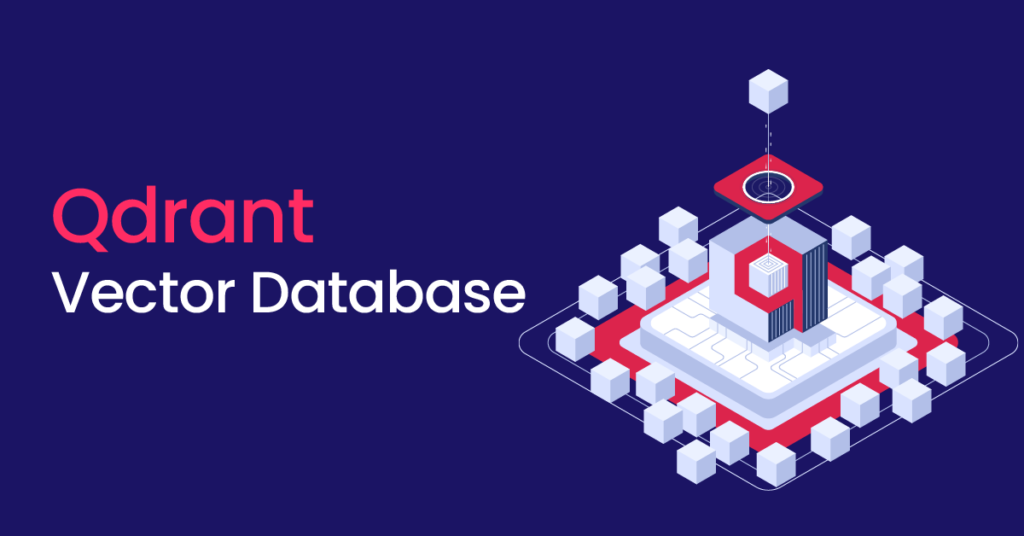
Qdrant notifies relevant authorities and/or the person concerned of any possible violations of data under the conditions of the Data Protection Laws.
Use Cases for Qdrant
Qdrant offers a range of use cases that leverage its powerful features for various applications:
1. Multitenancy and Custom Sharding:
- Qdrant supports multitenancy, allowing each tenant’s data to be isolated and accessible only by them. This feature is beneficial for scaling products like bank fraud-detection systems, e-commerce recommendation engines, or government services. Multitenancy enhances application performance and reduces hosting costs.
- Custom sharding in Qdrant enables users to specify a shard for each vector individually, providing control over data placement within the cluster. This feature is useful for optimizing data storage and retrieval based on specific criteria.
2. Semantic Search and Recommendation Systems:
- Qdrant is ideal for deploying applications that rely on matching embeddings produced by neural network encoders. It excels in semantic-based matching, faceted search, and other applications that require advanced vector similarity search technology.
- Use cases include building search engines, developing recommendation systems for various content types like news articles, songs, or skin conditions, creating question and answer systems, and implementing image comparison systems for different purposes.
3. Advanced Image Search and Business Logic Filtering:
- Qdrant’s vector search capabilities extend beyond text search to finding similar images, detecting duplicates, and even searching for images based on text descriptions. This feature is valuable for applications requiring image similarity comparisons and retrieval.
- The platform’s filters enable the application of arbitrary business logic on top of similarity search, allowing users to define complex search conditions like finding clothes under a certain price or artworks published within a specific timeframe. This flexibility makes Qdrant suitable for a wide range of use cases.
These use cases demonstrate the versatility and power of Qdrant in supporting diverse applications that rely on advanced vector similarity search technology and efficient data management strategies.
Ai applications that use Qdrant
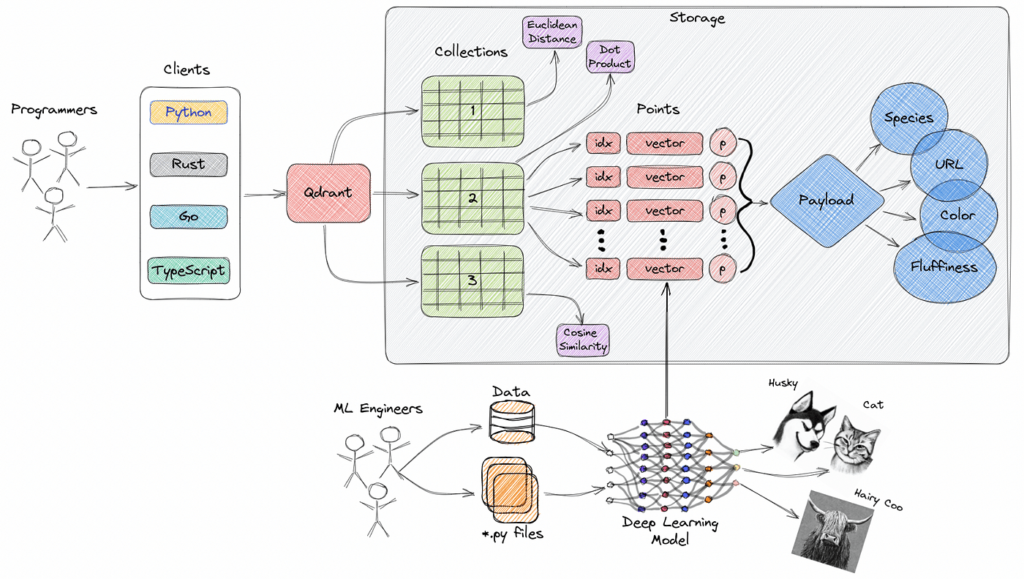
Here are some examples of AI applications that use Qdrant:
- Chatbots: Qdrant is used in chatbots to provide efficient and accurate solutions to specific queries, such as O Level Physics questions. It is also used to enhance the search functionality of e-commerce systems by integrating a semantic search feature.
- Semantic Search Engines: Qdrant is used in semantic search engines to understand customers in over 100 languages and help respond in legal documents. It is also used to discover the power of semantic search in government press releases with ease.
- Recommendation Systems: Qdrant is used in recommendation systems to provide content-based semantic recommendations with cohere-ai embeddings and qdrant vector search. It is also used to create smart assistant chatbots that educate teens with diabetes type 1 about their disease and how to deal with it.
- Legal Document Search Systems: Qdrant is used in legal document search systems to allow for efficient retrieval of legal documents that have been signed by mutual assent and can be relied upon in legal matters.
- Medical Q&A Systems: Qdrant is used in medical Q&A systems to provide accurate answers to user queries quickly and efficiently.
- Brand Monitoring Solutions: Qdrant is used in brand monitoring solutions to understand customers in over 100 languages and help respond in legal documents.
- AI-based Corporate Management Tools: Qdrant is used in AI-based corporate management tools to allow staff to always stay up to date with every part of the company’s ongoing work.
These examples demonstrate the versatility and power of Qdrant in supporting diverse AI applications that rely on advanced vector similarity search technology and efficient data management strategies.
What is the Pricing of Qdrant?
The pricing model for Qdrant varies based on different deployment options:
Service | Pricing | Features |
---|---|---|
Managed Cloud | $0 | – 1GB free forever cluster – Fully managed with central cluster management – Multiple cloud providers and regions (AWS, GCP, Azure) – Horizontal & vertical scaling – Central monitoring, log management and alerting – High availability, auto-healing – Backup & disaster recovery – Zero-downtime upgrades – Unlimited users – Standard support plan – Upgradable to premium support plan |
Hybrid Cloud | $0.014 per hour | – All benefits of Managed Cloud – Bring your own cluster from any cloud provider, on-premise infrastructure, or edge locations – Use Managed Cloud Central Cluster Management – Standard support plan – Upgradable to premium support plan |
Private Cloud | Custom (Price on request) | – All benefits of Hybrid Cloud – Deploy Qdrant fully on-premise for maximum control and data sovereignty – Use Managed Cloud Central Cluster Management or run the Central Cluster Management Interface in your own infrastructure, in the cloud, on-premise at the edge, even fully air-gapped – Premium support plan |
- Qdrant Cloud Pricing:
- Community: Free under the Apache-2.0 license.
- Managed Cloud: Starting from $25 per node per month billed hourly.
- Enterprise: Price on request, priced per managed cluster.
- AWS Marketplace Pricing:
- Qdrant Cloud usage unit pricing according to the cluster deployment is $0.01 per unit.
- The AWS Marketplace listing for Qdrant Cloud streamlines access for users relying on Amazon Web Services. Billing for Qdrant usage is processed through the AWS Marketplace, and the payment is carried out via the user’s AWS Account.
Free version of Qdrant
The free version of Qdrant, available under the Apache-2.0 license, includes the following features and offerings:
- Community Version:
- Free usage under the Apache-2.0 license.
- Access to Qdrant’s open-source vector database and vector search engine capabilities.
- Ability to build Minimum Viable Products (MVPs) on an open-source stack.
- Suitable for development and experimentation purposes without any cost associated.
- Qdrant Cloud Free Tier:
- Free tier on Qdrant Cloud for exploring the services without the need to provide credit card details.
- Allows for experimenting with around 1 million of 768-dimensional vectors, with the ability to store additional attributes with vectors.
- Offers flexibility to create several collections or keep multiple vectors per point, enabling the building of multiple small demos even on the free tier.
- Features:
- Access to Qdrant’s expanding features for neural network or semantic-based matching, faceted search, and other applications.
- Support for local deployment, on-premise setup, and Qdrant Cloud usage for development and testing purposes.
- Ability to attach your own infrastructure to Qdrant Cloud for building private environments tailored to specific needs
Benefits of Qdrant
Benefits of Qdrant | Limitations of Qdrant |
---|---|
– Speed and reliability | – Performance tuning required |
– Versatility | – Limited support for high-dimensional data |
– Advanced filtering capabilities | – Limited support for distributed deployment |
– Convenient API | – Limited support for real-time data ingestion |
– High-performance and scalable | – Limited support for advanced indexing and search capabilities |
– Enterprise-ready | |
– Advanced vector similarity search technology | |
– Efficient storage and retrieval of vector data | |
– Flexibility and advanced functionality | |
– Continuous improvement | |
– Community engagement | |
– Integration with existing systems |
Qdrant offers several benefits as a vector similarity search engine and vector database. Some of these benefits include:
- Speed and reliability: Qdrant is built using Rust, which provides fast and reliable performance for vector search operations.
- Versatility: Qdrant can be used for a wide range of applications, including neural network or semantic-based matching, faceted search, and extreme classification. It offers a range of demo projects, including semantic text search, similar image search, and e-commerce product categorization, demonstrating its versatility.
- Advanced filtering capabilities: Qdrant offers advanced filtering capabilities, allowing for the application of arbitrary business logic on top of similarity search. This makes it useful for complex querying and filtering of results.
- Convenient API: Qdrant provides a convenient API to store, search, and manage vectors, making it easy to integrate into existing systems and workflows.
- High-performance and scalable: Qdrant is designed for high-performance and scalability, with support for distributed deployment and SIMD hardware acceleration. This makes it suitable for large-scale vector search applications.
- Enterprise-ready: Qdrant is available as an open-source, cloud, and managed on-premise solution, making it suitable for enterprise-level applications.
- Advanced vector similarity search technology: Qdrant offers advanced vector similarity search technology, powering the next generation of AI applications.
- Efficient storage and retrieval of vector data: Qdrant enables fast retrieval of similar vectors, overcoming limitations of traditional text search.
- Flexibility and advanced functionality: Qdrant offers flexibility and advanced functionality, making it an ideal solution for projects requiring semantic-based matching and real-time querying.
- Continuous improvement: Qdrant’s development team continuously improves performance and adds new features, ensuring that it stays up-to-date with the latest advancements in vector database technology.
- Community engagement: Qdrant has a thriving community, allowing users to engage, share experiences, and contribute to its future direction.
- Integration with existing systems: Qdrant offers a solution for stand-alone usage or integration into existing systems, making it a versatile tool for any project.
What are the Limitations of Qdrant ?
Qdrant has several limitations when compared to other vector search engines. These limitations include:
- Performance tuning: Qdrant requires users to spend time tuning the engine to optimize performance, depending on the application. This can be challenging for users who are not familiar with the engine or do not have expertise in Rust.
- Limited support for high-dimensional data: While Qdrant is designed for high-dimensional data, it may not perform as well as other vector search engines for specific use cases that require extremely high-dimensional data.
- Limited support for distributed deployment: Qdrant offers potential for distributed deployment, but it may not be as seamless or scalable as other vector search engines that are specifically designed for distributed environments.
- Limited support for real-time data ingestion: While Qdrant is designed for real-time querying, it may not support real-time data ingestion as well as other vector search engines that are specifically designed for real-time data processing.
- Limited support for advanced indexing and search capabilities: While Qdrant offers advanced filtering capabilities, it may not support advanced indexing and search capabilities as well as other vector search engines that are specifically designed for machine learning applications.
It is important to note that Qdrant is continuously being developed and updated, and many of these limitations may be addressed in future releases. However, when comparing Qdrant to other vector search engines, it is important to consider these limitations and how they may impact the specific use case.
Alternatives of Qdrant
The alternatives to Qdrant are:
- Sphinx
- Lucene
- Apache Solr
- MkDocs
- YugabyteDB
- Milvus
- Searchkick
- AddSearch
- SearchStax
- Algolia
- Expertrec
- Wizzy
- Zilliz Cloud
These alternatives offer various features, such as different deployment modes, supported technologies, performance metrics, and pricing options. It is essential to consider these factors when choosing an alternative to Qdrant.
For example, Sphinx is a search engine designed for indexing database content, while Algolia is a powerful API platform for dynamic experiences that helps businesses maximize the speed of search and discovery. On the other hand, Expertrec allows you to add a custom search engine to your website/online store without any coding.
Market Value And User Base of Qdrant
The market value of Qdrant is estimated to be between $113 million and $170 million. Qdrant has raised a total of $37.79 million in funding over four rounds, with its latest funding round being a Series A for $28 million in January 2024.
The company’s valuation was $50 million in April 2023. The company has seen significant growth in the past year, with over 5 million downloads and adoption by Fortune 500 companies like Deloitte, Hewlett Packard Enterprise, Bayer, and more.
Qdrant’s Series A funding round raised $28 million, led by Spark Capital, with participation from existing investors Unusual Ventures and 42CAP. The funding will be used to further accelerate Qdrant’s growth and empower enterprises with cutting-edge vector search technology to build next-gen AI applications at scale.
What Is The Revenue Of Qdrant?
Qdrant generates less than $1 million in revenue, according to Owler Company Profile The exact revenue figure is not specified in the publicly available sources, but it falls below the $1 million mark.
Faqs of Qdrant
Here are some key FAQs about Qdrant based on the provided sources:
- What are the primary features of Qdrant?
- Qdrant is a vector similarity search engine that allows for search with full-text filters, prefix search, semantic search-as-you-type, and support for sparse vectors.
- It provides a convenient API to store, search, and manage vectors with an additional payload, making it suitable for neural network or semantic-based matching and faceted search.
- What does Qdrant plan to introduce in the future?
- Qdrant plans to introduce ColBERT and other late-interaction models, as well as fusion of multiple searches.
- What functionalities does Qdrant not plan to support?
- Qdrant does not plan to support non-vector-based retrieval or ranking functions like BM25, built-in ontologies or knowledge graphs, or query analyzers and other NLP tools.
- How does Qdrant handle data migration and storage format changes during updates?
- When deploying a newer version, Qdrant automatically migrates data to the newer storage format, and this migration is not reversible. Compatibility is guaranteed between consecutive versions, and data must be upgraded one version at a time.
- Can Qdrant scale down the underlying disk storage?
- Qdrant does not support scaling down the underlying disk storage, but manual intervention might be possible in some cases. However, this is not guaranteed, and downgrading a cluster is not supported.
- What is the recommended method for running Qdrant in production?
Updated Date: 25/04/2024